First Things First: Setting the Data Foundation before Diving into ML/AI
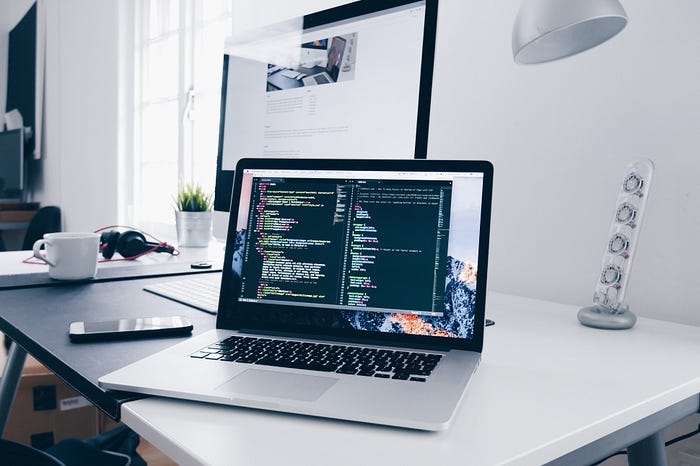
‘Data is the new gold’ is a common phrase in our day and companies are eager to jump on the Machine Learning/ Artificial Intelligence bandwagon, aiming to extract value from the vast amounts of data they’ve accumulated. But before venturing into the realm of ML/AI, there’s a fundamental question every company should ask: “Is our data in order?”
I have been opportune to work with a client that wanted to build some sort of predictive model. However, as we delved into the process, we encountered significant challenges related to data quality and siloed data repositories. This experience is not unique and highlights a common oversight many companies make — rushing into ML/AI without ensuring their data foundation is robust.
Why do I need a solid data foundation?
1. Garbage In, Garbage Out: Imagine planting an orange seed and expecting a mango tree. No matter how advanced your algorithms are, they cannot make sense of inconsistent, inaccurate, or incomplete data. Poor data quality leads to misleading results, which can result in flawed business decisions.
2. Time and Cost Efficiency: Data scientists spend a significant portion of their time cleaning and preparing data. If your data isn’t in good shape, this time skyrockets, delaying projects and increasing costs, and time, as they say, is money.
3. Siloed Data Hinders Holistic Insights: Data trapped in silos prevents a 360-degree view of operations and customers. Integrating data sources is fundamental to getting the most comprehensive insights.
What then should I do?
1. Data Auditing: Regularly review your data for quality and consistency. Identify any missing, outdated, or redundant information.
2. Implement Data Governance: Imagine playing football without any rules. Chaotic, right? Establish rules and protocols for data collection, storage, and management. This ensures standardization and reliability. Implementing a robust data governance framework cannot be over-emphasized.
3. Integrate Data Sources: Invest in tools and platforms (Enterprise Data Warehouse, Master Data Management, etc) that can consolidate data from different parts of the business. This not only aids in analytics but also ensures data integrity.
4. Educate & Train Staff: Ensure that everyone, from IT to marketing, customer service to executives understands the importance of data quality. A small error in data entry can have significant downstream effects. We all have a part to play in the quality of our data
In conclusion, while the allure of ML/AI and its benefits is undeniable, it’s essential to have a strong foundation. Companies should ensure their data infrastructure is robust and reliable before investing heavily in ML/AI endeavors. After all, a house built on a shaky foundation, no matter how beautiful, is bound to collapse. In the data-driven era, it’s the companies that prioritize data quality and infrastructure that will stand tall and gain a competitive edge.